Machine Learning meets Continuum Robotics
Case Study: Concentric Tube Continuum Robots
Concentric tube continuum robots (CTCR) are composed of multiple concentric, pre-curved super-elastic tubes that are rotated and translated relative to one another. Due to the elastic interactions between the tubes, highly non-linear behavior characterizes the mapping between task space and joint space of a CTCR. To reflect this interaction, model-based approaches utilize static models based on the theory of Cosserat rods and are formulated as a boundary value problem which is solved numerically. In favour of computation time, some physical phenomena, e.g. friction or tube tolerances, are commonly neglected in these Goldstandard physics-based modeling approaches. This model-based approach sufficiently represents the underlying mechanics, but has limitations in terms of accuracy as well as efficiency. An inversion of the model can only be realised numerically by iteratively solving the forward model at high computational expense. High computational expense is also characterising state of the art methods in computational design optimization and motion planning, while not exploring the complete parameter space and thus leading to suboptimal results. Learning-based models of concentric tube continuum robots have outperformed the Goldstandard Cosserat rod model with approximation errors below 1%!Model-based vs. Data-based Methods
The aim of this research programme is to leverage learning-based approaches for modelling, computational design, and motion planning for continuum robots. The long term vision is to enable technology transfer of these techniques to real-world applications of these robot, such as minimally invasive surgery, by focusing on the knowledge gaps for step change research. In the effort of achieving this, this research programme is planned around four objectives: - Define Data Representations - Investigate Deep Learning for Robot Modelling - Enable Task-Optimal Robot Designs by Reinforcement Learning - Explore Learning-based Motion Planning TechniquesFunding
- NSERC Discovery Grant (April 1, 2019 – March 31, 2025)
- NSERC Discovery Accelerator Supplements
- NSERC Discovery Launch Supplements (2019 - 2020)
Publications
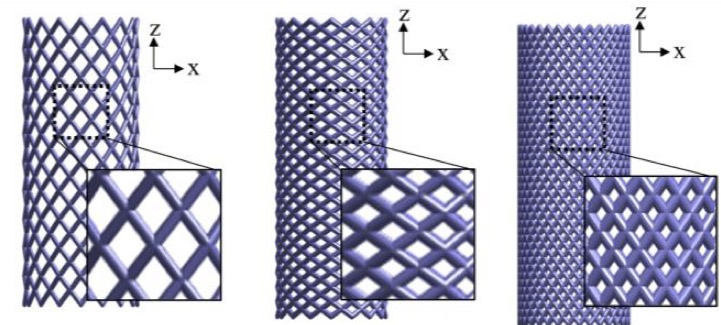
Design of Patterns in Tubular Robots Using DNN-Metaheuristics Optimization
International Journal of Mechanical Sciences, 251:108352, 2023.
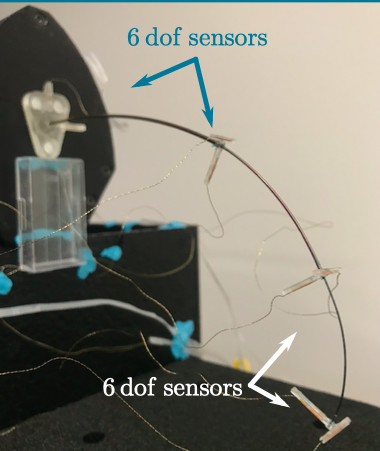
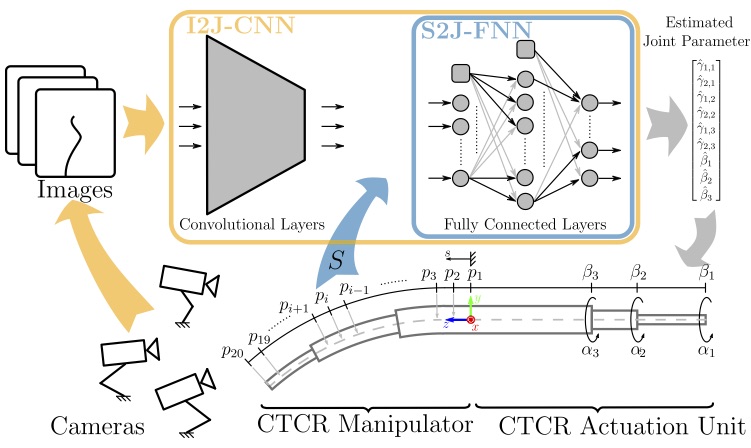
Learning-based Inverse Kinematics from Shape as Input for Concentric Tube Continuum Robots
IEEE International Conference on Robotics and Automation (ICRA), 2021.
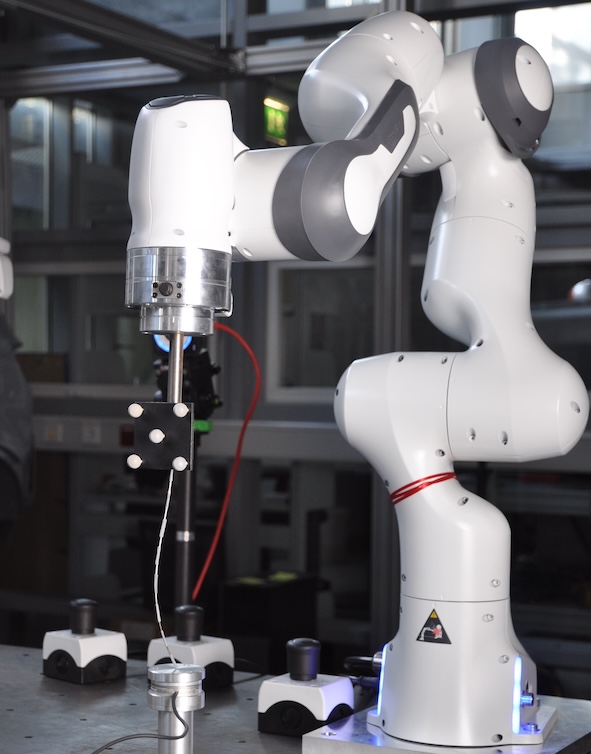
Estimating Tip Contact Forces for Concentric Tube Continuum Robots based on Backbone Deflection
IEEE Transactions on Medical Robotics and Bionics , 2 (4), pp. 619-630, 2020.
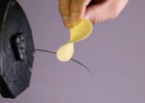
On the Merits of Joint Space and Orientation Representations in Learning the Forward Kinematics in SE(3)
Robotics - Science and Systems Conference, 10 pages, 2019.
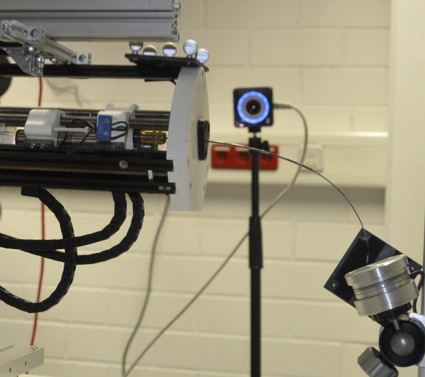
Towards Learning Force Sensing for a Concentric Tube Continuum Robot
Workshop on Open Challenges and State-of-the-Art in Control System Design and Technology Development for Surgical Robotic Systems, IEEE International Conference on Robotics and Automation, 2019.
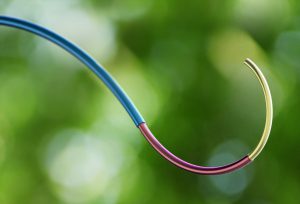
Learning the Forward and Inverse Kinematics of a 6-DOF Concentric-Tube Continuum Robot in SE(3)
IEEE/RSJ International Conference on Intelligent Robots and Systems, pp. 5125-5132, 2018.